BEST ML VISUALS
What Is Machine Learning? — A visual explanation.
9 curated images, interactive tools and flowcharts that explain machine learning.

To get a good grasp of machine learning, words are not enough. This article introduces you to machine learning using the best visual explanations I’ve come across over the last 5 years.
1. Machine learning finds patterns in data
Simply, machine learning finds patterns in data and uses them to make predictions.
Whenever you have large amounts of data and want to automate smart predictions, machine learning could be the right tool to use.

2. AI vs. machine learning vs. deep learning
Let’s get this out of the way first. What’s the difference between AI, machine learning, and deep learning? This chart from NVIDIA explains:
- Artificial intelligence is an academic discipline founded in the early 1950s.
- Machine learning is the study of algorithms that learn by experience. It’s been gaining momentum since the 1980s and is a subfield of AI.
- Deep learning is a newer subfield of machine learning using neural networks. It’s been very successful in certain areas (image, video, text, and audio processing).

So what term should you use?
- Short answer: It’s not so important.
- Longer answer: The term most used by practitioners in the field is “machine learning” — it’s more specific than AI.
“If it’s written in Python, it’s machine learning; if it’s written in PowerPoint, it’s AI.” 😅
3. The difference between ML and “normal” software
A machine learning algorithm is also just software. So then what’s the big difference?
3.1 The short answer
With “normal” software, we tell the computer what to do. With machine learning, we tell the computer how to figure out the answer itself, using the data we feed it.

3.2 The slightly longer answer
- Normal software is basically a set of rules, written by a human, intended to achieve a particular output.
- Machine learning software finds rules (patterns) on its own and tries to produce a certain output. It’s software that writes software. 🤯
Explained visually:

4. How do ML algorithms learn?
But how does machine learning find the right patterns in the data to make predictions?
The team from R2D3 managed to visualize how a machine learning model learns. The task: “Tell me whether an apartment is in New York or San Francisco.”
👉 Their interactive visualization of machine learning is nothing short of heroic. It’s technically accurate and beautiful.
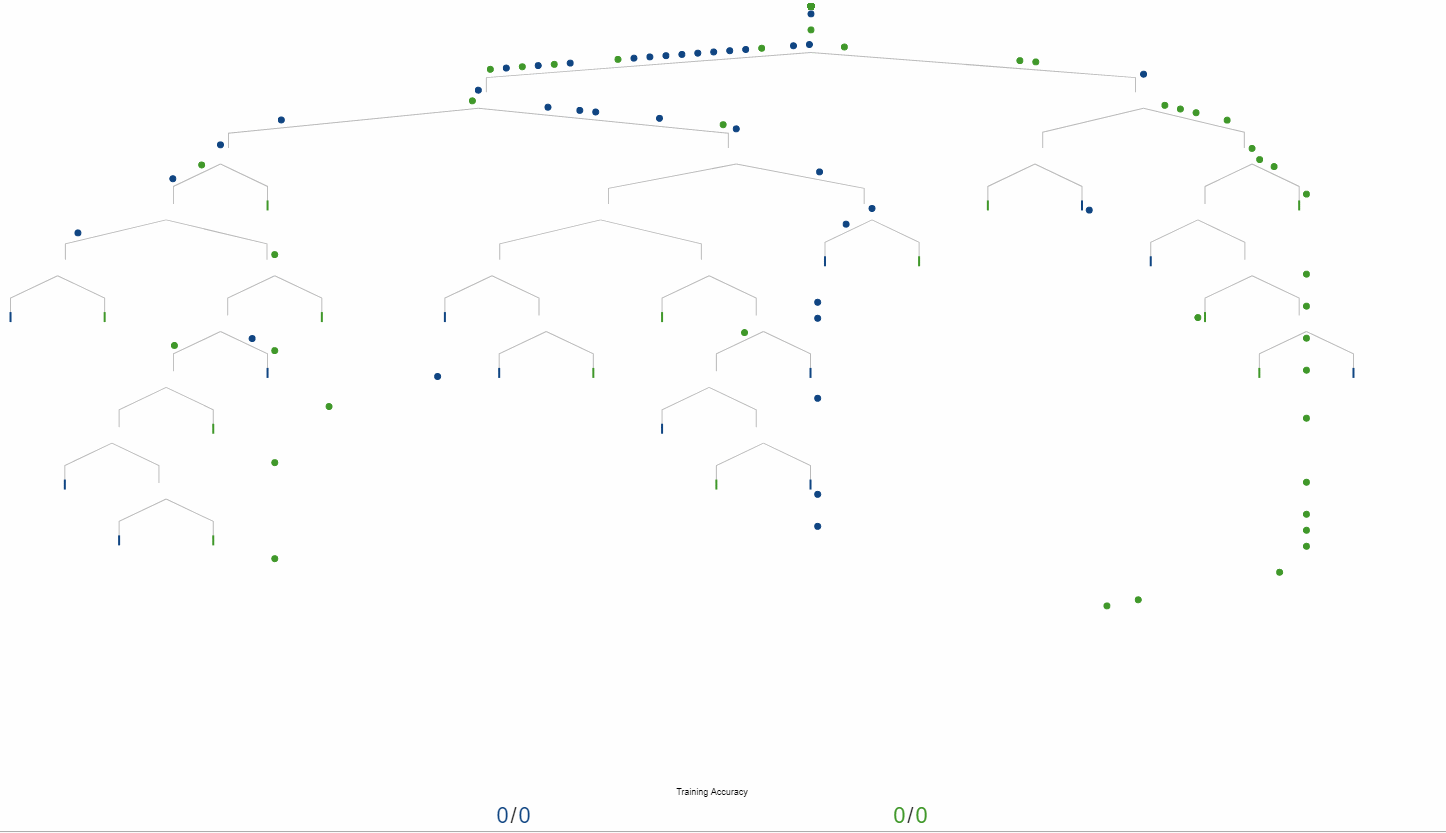
5. What about deep learning?
Google’s got you covered. Forget boring “network graphs.” Check out 👉 this live, interactive example of how a neural network learns.
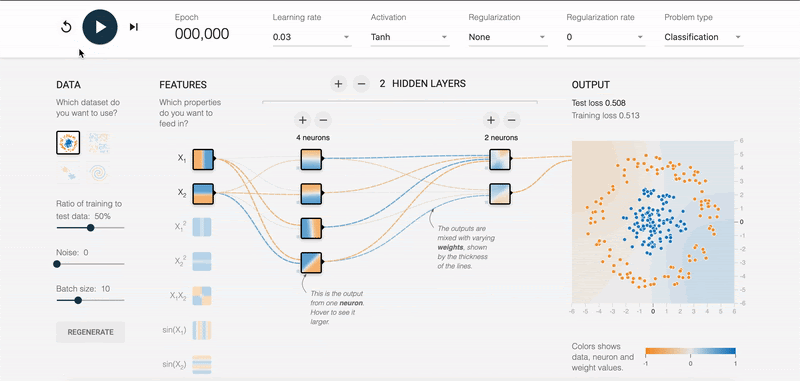
You can even modify the:
- Training parameters, meaning the configuration (nobs) of the algorithm;
- Neurons and layers, which define how complex the model can get;
- Task, so that the model learns to separate dots in a circle, a rectangle, or — the hardest task — a spiral.
For each model, you can watch how it gets better over time (test loss).
6. But how does a neural network work?
Neural networks are a bit more complex — but if you’re seriously interested, then there’s no better video to explain it than 👉 3Blue1Brown — What is a neural network, where Grant tells you how a neural network recognizes digits.
7. How do you tell whether it’s machine learning?
Here’s a quick and easy flowchart to help you figure out whether machine learning is behind what you’re seeing:

8. What are the elements of an ML solution?
The truth — machine learning is only a small part of every machine learning solution. Most of the work and code goes into the infrastructure around it:
- Data: collecting, verifying, analyzing, and preparing data for the algorithm;
- Infrastructure: deploying, hosting, and serving the machine learning model;
- Monitoring: orchestrating the workflows, monitoring and maintaining the model.

9. What ML algorithms are there?
We have many algorithms to choose from. They roughly fall into these categories:
- Classical learning: further split into supervised learning (predicting a number or category by learning from examples) or unsupervised learning (finding clusters in data without any guidance).
- Neural networks and deep learning: algorithms based on the principles of multi-layered perceptrons, which are very useful in making predictions with image, text, audio, video, and time-series data.
- Reinforcement learning: algorithms that work a bit like natural evolution, improving their behaviour in learning cycles to reap maximum rewards.
- Ensemble learning: algorithms that build lots of weak models and combine them to make a strong model.

Summary
These visuals should give you a good grasp of all the basic machine learning concepts. To dive deeper into how to use machine learning in your projects, check out our Guide to machine learning: What project managers need to know.
Let me know if there’s something important missing: markus@datarevenue.com.
Originally published at https://www.datarevenue.com.