Machine Learning In Web Analytics — Has Google Analytics Changed for Better?
Google Analytics 4 was recently launched which has advanced Machine learning capabilities. Will ML rule the world of web analytics in the next 5 years?

Businesses have been trying to understand customers and their buyer’s journey in a better way to drive better conversions and increase lifetime value. And, in today’s world, when the majority of a buyer’s journey is on the web, web analytics has become more significant than ever.
Web analytics put together with Machine learning capabilities can truly be remarkable for businesses. And Google Analytics 4 has strolled a mile into this lane.
In this article, I’ll talk about Google Analytics 4 and how have the newly introduced Machine Learning capabilities changed Analytics for the better.
But before we get into that, let’s review how Machine Learning has and can revolutionalize web analytics!
Machine Learning in Web Analytics
Data gathered is of no use unless it can be segregated and processed. With the increasing number of users on the web, the average traffic across websites is constantly increasing.
Analyzing these huge sets of data was a pain until Big Data Analytics (BDA) using machine learning came into the picture.
Big Data Analytics (BDA)
A research paper titled — “A survey towards an integration of big data analytics to big insights for value-creation” mentions seven characteristics of Big Data Analytics as below:
The seven characteristics of BDA include “Volume” (support of very high data volumes), “Velocity” (fast analysis of data streams), “Variety” (support for diverse kinds of data), “Veracity” (support for high data quality), “Value” (the value of the insights and benefits), “Variability” (support for constantly changing), and “Valence” (support of connectivity in data).
Having said that, two of the most common data analytic algorithms, as most of us know, are Supervised ML Algorithms and Unsupervised ML Algorithms.
For a few who don’t know the difference between these two, here’s what supervised ML and Unsupervised ML algorithms are, in a nutshell.
Unsupervised Methods/algorithms are self-learning models that are mostly used to discover undetected information/patterns.
Supervised ML algorithms, on the other hand, take a known input data & known output for this data and it uses this information to train a model for generating reasonable predictions for a completely new data set.
These two, combined, have completely changed the way businesses use web analytics and for the better.
The importance of Big Data Analytics in using web analytics in the decision-making aspect of businesses is increasing by each day. And this correspondingly is increasing the urgency of the application of Machine Learning algorithms in web analytics for value creation and organic business growth.
Google Analytics & Machine Learning
Google Analytics is the leader in the field of web analytics and the two major types of data, Analytics collects, are — User Properties/Dimensions and Events.
Businesses have been using these data for optimizing their websites’ data by understanding their users in a better way.
In the User Dimensions category, a few types of data that is collected by Google Analytics are — Age, Gender, Country, Device Model/Category, etc. A few types of events that are collected by Google Analytics are — search, select_content, sign_up, click, file_download, first_visit, etc.
Millions of small and large scale businesses use Google Analytics to understand their customers in a better way and create better user experiences for them.
Using Machine Learning in web analytics is definitely not a new thing but the way Machine Learning is now being used in Google Analytics 4 is new!
Over the years, people have used Machine Learning capabilities in web analytics to understand web traffic in a better way.
For instance, we have seen earlier on Towards Data Science — Send Google Analytics Hit Level Data to BigQuery and Automate Data Import to Google Analytics.
Besides these, we have seen how supervised or unsupervised clustering can help us understand web traffic in a more comprehensive way.
If you are unfamiliar with how to use clustering — a Machine Learning technique to understand web traffic, here’s an article by Russ Thompson that will help you get the basic idea.
But when we are talking about using Machine Learning in web analytics, you should know that every machine learning model needs few elements for maximum impact:
- The Question that you need your ML model to answer.
- Preparation of data to feed into your model. People prefer to use R to gather web analytics data from Google Analytics or any other source.
- Assessment of output from your ML model. While you may understand the data your Machine Learning model in web analytics produces, but you’ll have to go for data visualization to make it comprehensible for the management as well as non-technical team members.
- Utilizing the data for better ROI. Improving business ROI is one of the core reasons to use Machine Learning in web analytics.
But what if Google Analytics itself starts using Machine Learning and help you make the most out of it by presenting insights using data in a comprehensible format better than ever before? Well, GOOD NEWS — Google Analytics 4 is doing that!
Google Analytics 4: How Has Machine Learning Changed Google Analytics?
In October 2020, Google made Google Analytics 4 available in the public domain. However, the beta version of something similar was released in July 2019.
The initial idea was to combine app and web analytics to inspire better ROI in marketing and businesses, in general.

After testing the usability of Machine Learning in Google Analytics for more than 1 year, Google finally launched Google Analytics 4, known as App+Web Properties during the beta period.
Before Analytics 4, you could ask questions like “Which products had over 100 purchases in the last month?” and Analytics Intelligence would generate results using its ML capabilities.
But here’s what’s new in Google Analytics 4 after the introduction of Machine Learning.
Monitor Traffic in Real-Time
You can now ask questions in all supported language to generate results in real-time. You can use the property “Realtime” to get Analytics data from the last 30 minutes. For instance, you can monitor traffic to your website/app from a one-day promotion.
You can also monitor the immediate effects of a campaign you are running or how recently made changes on your website or app is impacting them.
Search for Instant Answers
You can ask for Analytics data in natural languages to get instant answers in Google Analytics 4. You can be specific about a property, metric, time frame, or dimension.
For instance, you can search for “conversions last week from Arizona” or “mobile users from the United States in the previous week” to get Reports, Data, Insights, and more.
Cross-Platform Analysis
One of the key highlights of “App+Web properties” and now Google Analytics 4 is its ability to show you insights by combining the data of your web and app users. Using Machine Learning capabilities, Google Analytics 4 gives you instant data visualization based on any property you select — metrics, dimensions, etc.
You can define as many as 10 steps in your user funnel using Google Analytics 4 and clearly visualize how your users are moving in or bouncing off at each step. This will help you find answers to what is working and what is not for your web or mobile app users!
Smart Alerts
The new Google Analytics 4 alerts you if your website or app sees a significant increase/decrease in users or a changing trend in your web/app data using Machine Learning capabilities.
These smart alerts will help you take any instant action as a result of a sudden spike or sudden decline in the number of users. Besides, the new Analytics 4 can help you anticipate your user actions well in advance. For instance, it can let you predict your revenue from a particular segment of users — churn probability.
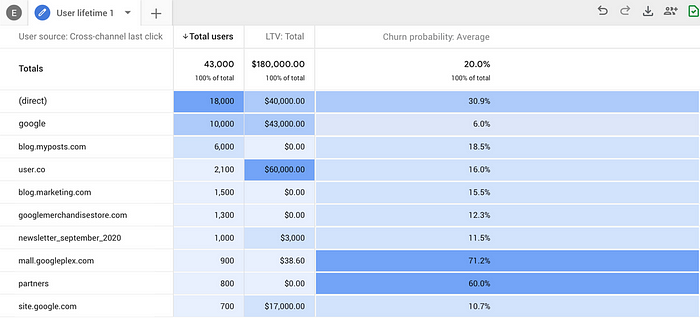
Google Signals
While Google Signals are not new, turning Google Signals on in Google Analytics 4 can really help you improve your marketing ROI and take steps for real-time lead generation.
For instance, using Google data from the users who have switched on Ads personalization, Analytics 4 generates reports with more accurate user counts when Google Signals are turned on.
These were just a few things introduced/changes in the new Google Analytics 4 after the introduction of Machine Learning capabilities. Having said this, the introduction of ML in Google Analytics is just the beginning.
Machine Learning has a significant role to play in the future of Google Analytics as well as web analytics, in general.
Role of Machine Learning in Future of Web Analytics
Did you know that Microsoft Clarity — the Web Analytics Tool by Microsoft became publicly available in October 2020?
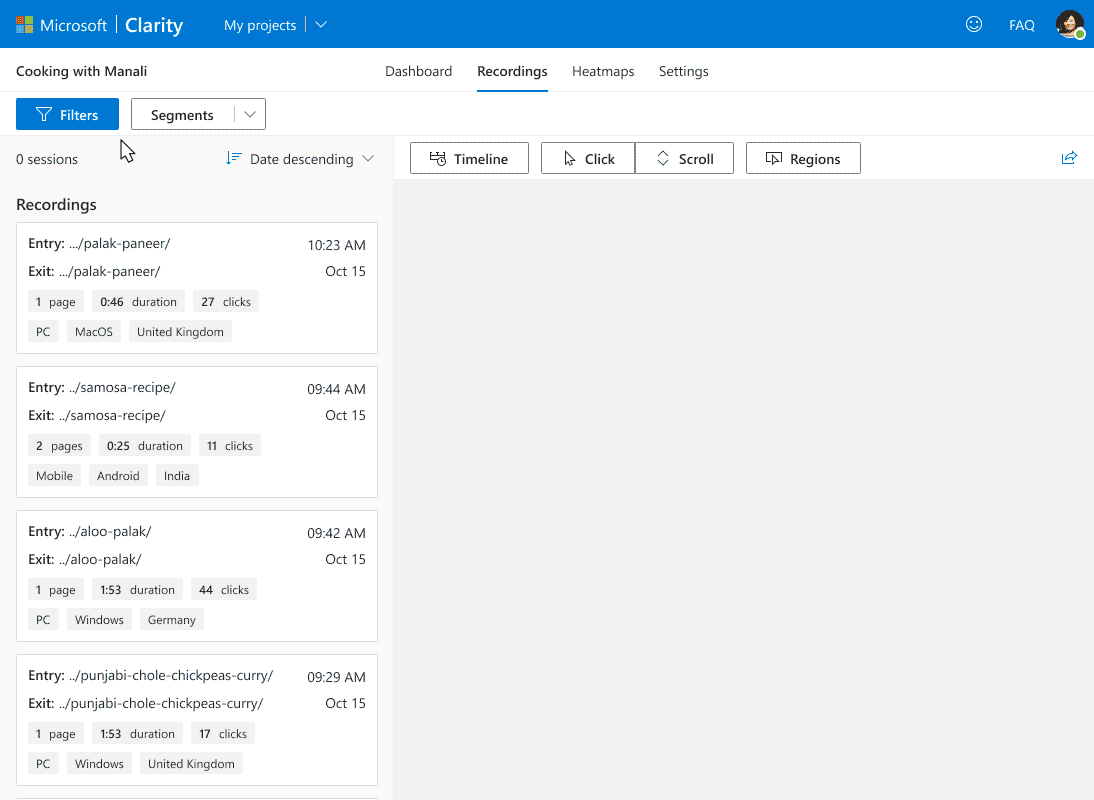
While a comprehensive comparison of Google Analytics 4 and Microsoft Clarity is yet to unveil, it is safe to say that Analytics 4 outstands Clarity given its capability to analyze app data as well.
Having said that, even Microsoft Clarity has added Machine Learning capabilities that allow its users to filter website data and session recordings using parameters such as “excessive recordings”, “dead clicks”, etc.
Machine Learning in Web Analytics is definitely the future and it looks bright. With new advancements in Natural Language Processing, like Google’s BigBird, Machine Learning can definitely revolutionize how we see and use web analytics today.
The good thing about the inclusion of advanced Machine Learning and Artificial Intelligence in web analytics, officially, by Google and Microsoft is that it will make it simpler to execute & measure campaigns for marketers &
businesses.
Besides, it also will help reduce marketing costs for businesses by optimizing ROI in real-time as well as eliminating the need to use third-party AI/ML tools for smarter web analytics.